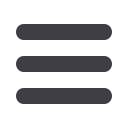
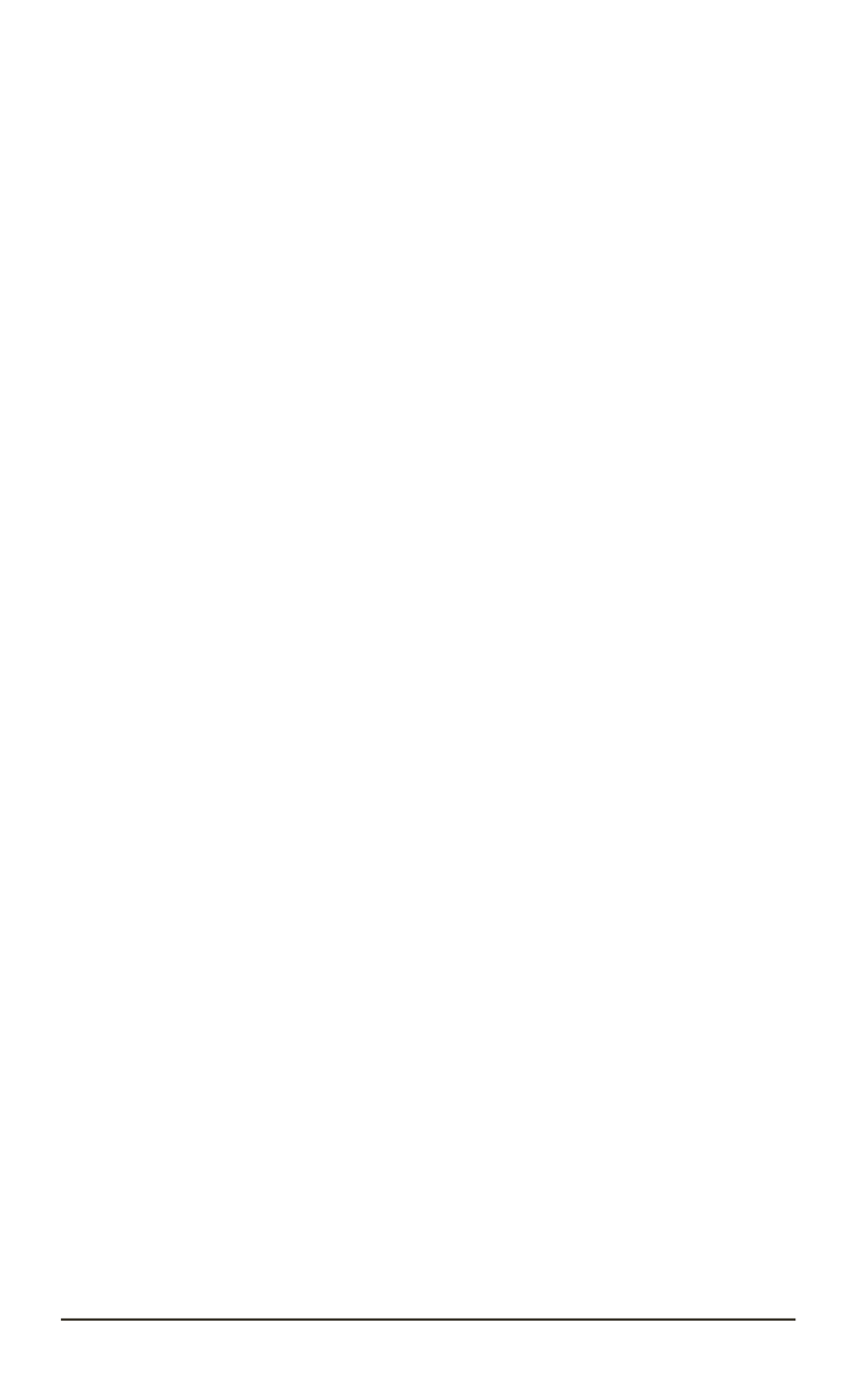
В практических компьютерных приложениях рассмотренный ме-
тод может быть полезен для организации и упорядочения аудиодан-
ных (например, для автоматического создания аудиоархивов), для ана-
лиза и визуализации сходства в характеристиках речи при проведении
различных исследований (идентификация человека по голосу, обнару-
жение похожих голосов и т.д.).
ЛИТЕРАТУРА
1.
Tzanetakis G.
,
Cook P.
Musical genre classification of audio signals // IEEE
Transactions on Speech and Audio Processing. 2002. Vol. 10. P. 293–302.
2.
Guo G.
,
Li S.Z.
Content-based audio classification and retrieval by support vector
machines // IEEE Transactions on Neural Networks. 2003. Vol. 14. P. 209–215.
3.
Li T.
,
Ogihara M.
,
Li Q.
A comparative study on content-based music genre
classification // SIGIR03. 2003. P. 282–289.
4.
Bagci U.
,
Erzin E.
Automatic Classification of Musical Genres Using Inter-Genre
Similarity // IEEE Signal Processing Letters. 2007. Vol. 14. P. 521–524.
5.
Toward
multi-modal music emotion classification / Y.H. Yang et al. // Proceedings of
the 9th Pacific Rim Conference on Multimedia: Advances in Multimedia Information
Processing. 2008. P. 70–79.
6.
Zlatintsi A.
,
Maragos P.
Multiscale fractal analysis of musical instrument signals
with application to recognition // IEEE Transactions on Audio, Speech and Language
Processing. 2013. Vol. 21. P. 737–748.
7.
McFee B.
,
Barrington L.
,
Lanckriet G.R.G.
Learning content similarity for music
recommendation// IEEE Transactions on Audio, Speech and Language Processing.
2012. Vol. 20. P. 2207–2218.
8.
Predictability
of music descriptor time series and its application to cover song
detection / Y. Serra et al. // IEEE Transactions on Audio, Speech and Language
Processing. 2012. Vol. 20. P. 514–525.
9.
Manders A.J.
,
Simpson D.M.
,
Bell S.L.
Objective prediction of the sound quality of
music processed by an adaptive feedback canceller // IEEE Transactions on Audio,
Speech and Language Processing. 2012. Vol. 20. P. 1734–1745.
10.
Downie D.
The music information retrieval evaluation exchange (2005–2007):
A window into music information retrieval research // Acoustical Science and
Technology. 2008. Vol. 29. P. 247–255.
11.
Casey M.
et al. Content-based music information retrieval: Current directions and
future challenges // Proceedings of the IEEE. 2008. Vol. 96. P. 668–695.
12.
George J.
,
Shamir L.
Computer analysis of similarities between albums in popular
music // Pattern Recognition Letters. 2014. Vol. 45. P. 78–84.
13.
Wndchrm
— an open source utility for biological image analysis /
L. Shamir et al. // Source Code For Biology And Medicine. 2008. URL:
http://www.scfbm.org/content/3/1/13(дата обращения: 01.10.2014).
14.
Shamir L.
Evaluation of face datasets as tools for assessing the performance of
face recognition methods // International Journal of Computer Vision. 2008. Vol. 79.
P. 225–230.
15.
WND-CHARM
: Multipurpose image classification using compound image
transforms / N. Orlov et al. // Pattern Recognition Letters. 2008. Vol. 29. P. 1684–
1693.
16.
Deshpande H.
,
Singh R.
,
Nam U.
Classification of music signals in the visual
domain // Proceedings of the COST G-6 Conference on Digital Audio Effects
(DAFX-01). 2001. Vol. 1. P. 1–10.
136 ISSN 0236-3933. Вестник МГТУ им. Н.Э. Баумана. Сер. “Приборостроение”. 2015. № 3